Regularized Semi-paired Kernel CCA for Domain Adaptation
Manual labeling of sufficient training data for diverse application domains is a costly, laborious task and often prohibitive. Therefore, designing models that can leverage rich labeled data in one domain and be applicable to a different but related domain is highly desirable. In particular, domain adaptation or transfer learning algorithms seek to generalize a model trained in a source domain (training data) to a new target domain (test data). This paper introduces a regularized semi-paired kernel canonical correlation analysis formulation for learning a latent space for the domain adaptation problem. The optimization problem is formulated in the primal-dual least squares support vector machine setting where side information can be readily incorporated through regularization terms. The proposed model learns a joint representation of the data set across different domains by solving a generalized eigenvalue problem or linear system of equations in the dual. The approach is naturally equipped with out-of-sample extension property, which plays an important role for model selection. Furthermore, the Nyström approximation technique is used to make the computational issues due to the large size of the matrices involved in the eigen-decomposition feasible.
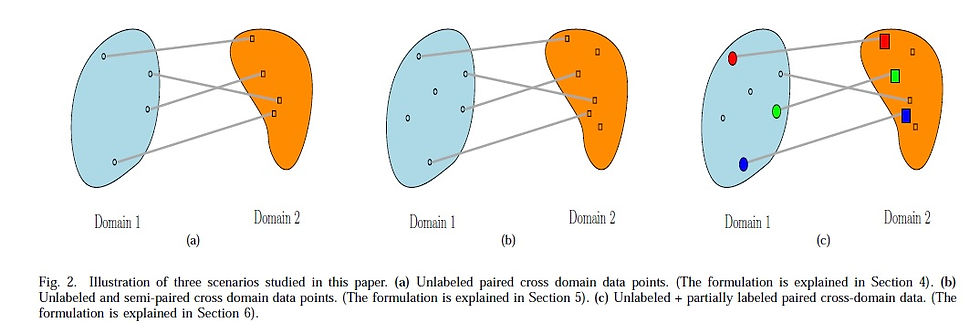

S. Mehrkanoon, J.A.K. Suykens, “Regularized Semi-Paired Kernel CCA for Domain Adaptation”, Accepted for publication in IEEE Trans. Neural Netw. Learning Syst, 2017. [PDF]